Data Inaccuracy is Risky in Financial Services
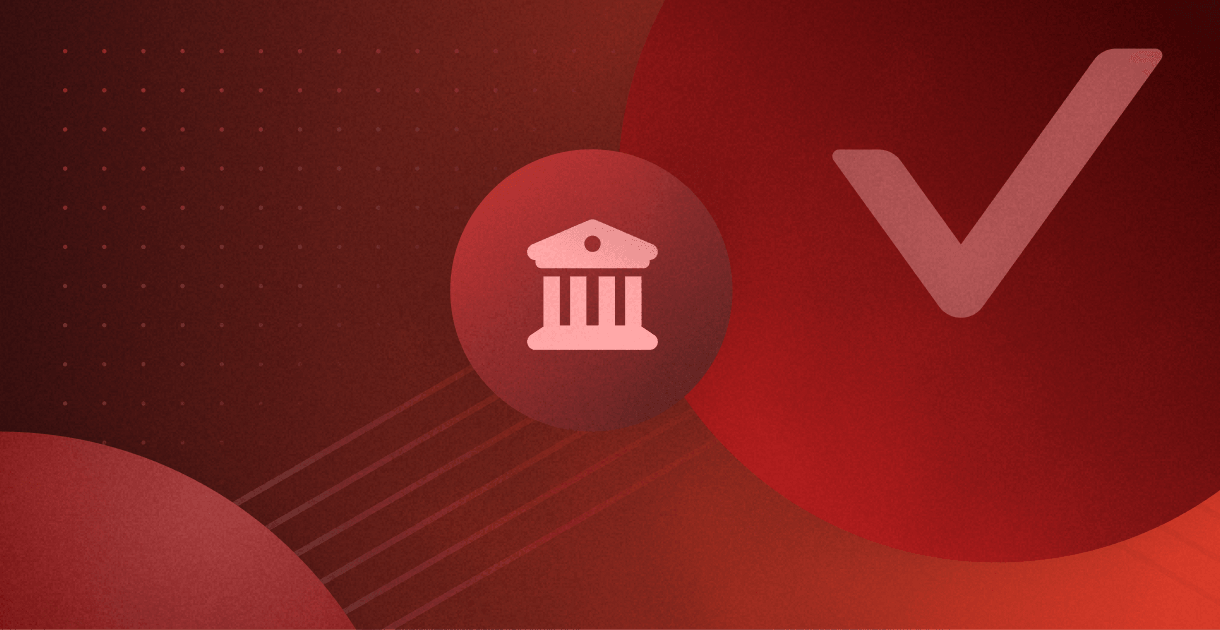
For financial services companies, inaccurate or outdated data can cause big problems.
For financial services companies, inaccurate or outdated data can cause big problems. A minor error in a single customer’s information could quickly spiral into a massive issue, affecting countless transactions and interactions. This is why financial data quality management is so important—bad data influences many areas of the business.
The consequences of data inaccuracies can be severe, impacting customer experience, revenue, and even regulatory compliance. In this article, we’ll explore the benefits of maintaining good financial data quality, address the data quality challenges faced by financial service companies, and offer insights on implementing a robust data quality process to safeguard a financial organization’s reputation and financial performance.
The Benefits of Financial Data Quality
By implementing a comprehensive data quality strategy, financial service providers decrease risk, increase productivity, and drive business growth. Here are some of the benefits businesses can expect from a properly implemented data quality initiative:
Enhanced Customer Experience. Accurate financial services data enables institutions to offer personalized and efficient services, resulting in a better customer experience. In contrast, data inaccuracies can lead to miscommunication, incorrect transactions, and ultimately, dissatisfied customers who may take their business elsewhere.
Improved Regulatory Compliance. Financial institutions operate in a highly regulated environment, and compliance with these regulations often hinges on the quality of data. By maintaining accurate financial services data, organizations can better detect and prevent financial crimes, reducing the risk of penalties, reputational damage, and costly legal battles.
Streamlined Operations. Clean, accurate data helps financial institutions optimize their processes, reduce errors, and decrease the time spent on manual data reconciliation. As a result, organizations can focus on their core business functions and achieve operational excellence.
Informed Decision-Making. Reliable data is the foundation for effective decision-making in the financial services industry. With accurate information at their fingertips, business leaders can make informed strategic decisions, identify growth opportunities, and accurately assess risk.
Increased Revenue and Cost Savings. Data inaccuracies can lead to lost revenue and increased costs, as organizations must devote resources to fixing errors and addressing customer complaints. By maintaining financial data quality management strategies, financial institutions can avoid these pitfalls.
Common Data Quality Challenges in Financial Services
Financial institutions face a myriad of data quality challenges daily, which can have far-reaching consequences for their operations and reputation. Here is a list of common challenges faced in the industry:
Data Inconsistency. Financial services data inconsistency arises when different data sources provide conflicting information, causing confusion and uncertainty. This may occur because of manual errors, outdated records, or system glitches, ultimately leading to inaccurate decision-making and increased operational risks. As financial institutions collect more data from more sources, these inconsistencies become a growing problem.
Data Fragmentation. Financial institutions often rely on multiple systems and databases to store and manage their data. As a result, data can become fragmented and siloed, making it difficult to obtain a holistic view of customers, transactions, and financial activities, impairing effective analysis and decision-making. Combining separate databases is not a small project. Merging data requires data to be in the same format and minor differences, such as a name spelling error, can cause duplicated information. Financial companies must use software specifically designed to resolve data differences and merge data from multiple sources.
Incomplete Data. Missing or incomplete data can significantly hinder a financial institution’s ability to find patterns, assess risks, and make informed decisions. Incomplete data can even lead to regulatory penalties, as financial institutions are required to keep comprehensive records to follow industry regulations. Debt information missing from a loan application, for example, can lead to lenders offering loans to borrowers whose correct debt to income ratio would otherwise prevent them from qualifying. Eventually, failed repayments can cause financial loss.
Data Decay. Over time, customer data can become outdated or inaccurate because of changes in personal circumstances, such as moving to a new address or changing phone numbers. This data decay can impact marketing efforts, customer service, and risk assessments, leading to missed opportunities and increased costs.
One common situation affects financial institutions who encourage customers to receive statements and bills online. With no regular statement mailings, the bank continues to process customer transactions, unaware the customer has moved multiple times. When at some point regulations force the bank to send physical documents through the mail, they are returned as undeliverable. This initiates an expensive research activity to update customer data with current address information and remail the documents.
The High Cost of Data Inaccuracy in Financial Services
Data quality issues cost financial institutions an average of $15 million per year, according to a Gartner report from back in 2017. The impact of poor data quality continues to be a concern for financial service companies today. The financial impact is likely greater than it was in 2017.
Mistakes in customer data, such as outdated addresses or incorrect phone numbers, can have a ripple effect on an organization’s efficiency and bottom line. Left unchecked, these errors can quickly multiply, causing a detrimental impact on customer experience, interactions, and transactions.
Inaccurate data can lead to increased costs and lost revenue for financial institutions. The consequences of a single data error could include missed opportunities for marketing and sales, incorrect risk assessments, and even regulatory penalties. The stakes are high, and the costs can be significant if not addressed promptly.
Financial services companies must prioritize data quality and implement comprehensive strategies to guarantee the accuracy and integrity of their data. A well-rounded approach to data quality can help organizations to detect and prevent financial crimes, protect their reputations, and safeguard the broader financial system. In the end, clean and accurate data is the foundation for effective decision-making, risk analysis, and customer satisfaction in the financial services industry.
The Role of Data Quality in Identifying and Preventing Financial Crimes
Financial crimes are a persistent threat to financial institutions and their customers. Maintaining data quality within financial systems is crucial for detecting and preventing nefarious activities. Data quality plays a vital role in identifying and mitigating financial crimes.
Accurate Data for Monitoring and Detection. High-quality data is essential for effective monitoring and detection of suspicious transactions. Correct customer information, such as names, addresses, and transaction histories, allows financial institutions to recognize patterns and flag potentially fraudulent activities. Inaccurate data, on the other hand, can lead to false alarms or missed red flags, compromising the integrity of anti-fraud measures.
Compliance with Regulatory Requirements. Financial institutions must adhere to strict regulatory requirements, such as anti-money laundering (AML) and know your customer (KYC) policies. Accurate and complete customer data is crucial for meeting these obligations, as regulators expect financial institutions to demonstrate robust due diligence processes. Inaccurate data can cause non-compliance, leading to hefty fines and reputational damage.
The role of data quality strategies shouldn’t be underestimated. As this article from Forbes highlights, data quality issues can lead to compliance violations and regulatory penalties.
Customer Trust and Reputation. Protecting customers from financial crimes is essential for maintaining their trust and loyalty. Precise data helps financial institutions recognize criminal activities more effectively, enhancing customer experience and protecting their assets. A strong reputation for safeguarding customers can also attract new business and improve the overall brand image.
Efficient Risk Management. Financial institutions need to assess and manage risks associated with financial crimes. True data allows for better risk analysis, enabling organizations to highlight areas of vulnerability and apply necessary controls. Inaccurate data can lead to incorrect assessments and increased exposure to financial crimes, ultimately impacting profitability.
Key Components of an Effective Financial Data Quality Management Strategy
Financial institutions constantly juggle a myriad of challenges, and data quality management is one of the most critical aspects to ensure a smooth and efficient operation. By implementing a comprehensive data quality management strategy, organizations can effectively mitigate financial risks, enhance regulatory compliance, and protect their customers, as well as their reputation.
A well-rounded approach encompasses several key components: regular data audits, ongoing data validation, data governance policies, data cleansing, and employee training. By incorporating these critical elements, financial institutions can reduce data inaccuracies, mitigate risks, and enhance overall performance. They will safeguard their business and customers from the negative consequences of poor data quality.
To achieve these objectives, financial institutions should adopt 10 best practices:
- Clearly Define Data Quality Goals: Start by defining data quality goals and aligning them with the financial institution’s business objectives. Identify the specific data quality dimensions that are most critical to the organization, such as accuracy, completeness, consistency, timeliness, and validity.
- Establish Data Quality Standards: Develop clear and measurable data quality standards that define the acceptable levels for each data quality dimension. These standards will serve as benchmarks for evaluating and monitoring the quality of the financial data.
- Data Profiling and Assessment: Conduct a comprehensive data profiling and assessment process to understand the current state of the data. locate data sources, assess data accuracy, pinpoint data gaps, and evaluate data lineage and data transformation processes.
- Implement Data Validation and Cleansing: Create data validation rules to detect and correct errors, inconsistencies, and outliers in the financial data. Use automated data cleansing techniques to standardize formats, remove duplicates, and rectify data anomalies.
- Data Governance Framework: Carry out a robust data governance framework to confirm accountability and ownership of data quality processes. Define roles and responsibilities, establish data stewardship, and create policies and procedures for data management, data access, and data security.
- Data Integration and Consolidation: Ensure proper integration and consolidation of data from various sources to minimize data silos and inconsistencies. Implement data integration tools and techniques to streamline the data aggregation process and improve data accuracy.
- Regular Data Monitoring and Reporting: Institute ongoing data monitoring and reporting mechanisms to assess the quality of the financial data continuously. Employ data quality dashboards and reports to track data quality metrics and document any issues or trends that need attention.
- Data Quality Training and Awareness: Arrange for comprehensive employee data quality training. Foster a data-driven culture by promoting awareness of the importance of data quality and its impact on decision-making.
- Data Quality Measurement and Metrics: Define key performance indicators (KPIs) and metrics to measure the effectiveness of the data quality process. Regularly monitor and report on metrics to find areas of improvement and track progress.
- Continuous Improvement: Data quality is an ongoing process, so it’s essential to evaluate and continuously improve data quality initiatives. Regularly review and update data quality standards, refine validation rules, and incorporate feedback from data users to enhance the overall data quality process.
Maintaining high data quality is crucial for financial services organizations to safeguard customer satisfaction, avoid regulatory penalties, and protect the financial system. By implementing a comprehensive data quality strategy, these institutions can effectively decrease risks, enhance their decision-making processes, and ultimately drive business growth.
Now is the time for financial institutions to concentrate on data quality, invest in tools and processes, and foster a culture of data accuracy and integrity. A proactive approach to financial data quality management will not only ensure a more reliable and secure financial environment, but also pave the way for long-term success in this ever-changing industry.
Top-level financial institutions rely on data quality tools and support from Firstlogic to administer their data management strategies. Our Firstlogic Data Quality IQ Suite® and SAP’s Data Services Platform allows these organizations to stay on top of ever-changing data management challenges and risks.