What is Data Quality? Definition and Frequently Asked Questions
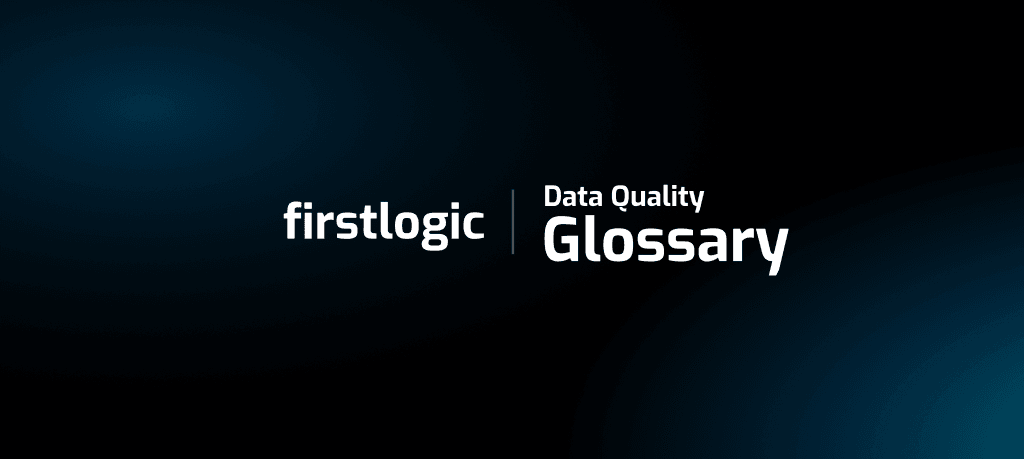
Data Quality: Definition
Data Quality is the measure of the suitability of a data set for its intended business role. In a business context, data quality is specifically measured for data quality characteristics including accuracy, completeness, and consistency.
FAQs
What is Data Quality?
Data quality refers to the degree to which data is accurate, complete, and consistent. High-quality data is necessary for organizations to make informed decisions and to effectively use data-driven technologies such as artificial intelligence and machine learning. Data quality is ensured through the implementation of procedures for verifying and cleaning data, as well as through the establishment of standards for data storage and formatting. Remember, Ensuring data quality is an ongoing process that requires regular monitoring and maintenance.
What are the dimensions of Data Quality?
Some of the most important dimensions of data quality to consider are:
Dimension | Definition | Addresses the question |
---|---|---|
Accuracy | How well a data set reflects reality “on the ground”. | Is the data free of mistakes? |
Comprehensiveness | Also known as data completeness. The characteristic of a dataset to | Is all the data present as required by the applications that use it? |
Consistency | The ability for a dataset to retain its value as it moves between data systems. | Is the value of the data the same across all systems, and does the data remain the same when moving from one system to another? |
Timeliness | The speed at which an organization’s data can be accessed for business operations. Related to data accessibility. | Is your data available when you need it, without delays that impact business operations? |
Definition | Also known as data validity. The characteristic of a dataset to conform to a set of business rules and agreed-upon standards within a business operation. | Is data in a specific format, does it conform to business rules? Is the meaning of the data clear, or is it open to interpretation? |
Uniqueness | The characteristic of a dataset to contain no duplicate data, in order to avoid operational errors. | Is this the only instance in which this data field appears in the database? |
Other characteristics, or dimensions, to consider include data granularity, data precision, data accessibility, data relevancy, and data currency.
How to improve data quality?
To improve data quality, a data-driven companies should establish a data quality process throughout their operation. This is often done using data quality management software with the help of a data quality expert consultant or a specialized in-house data team. The main focus of a data quality process is to ensure that the data is concise, consistent, and unambiguous. This typically involves standardizing, combining, and updating data, such as customer postal addresses. For example, technicians may verify that the addresses meet USPS standards and update addresses of customers who have moved.
An important aspect of establishing data quality likely involves reformatting data files to ensure consistency, such as ensuring that names are always stored in the same format. Data stewardship and data governance roles should be assigned, and these teams made responsible for establishing enterprise-wide field name conventions and ensuring that others in the organization follow these conventions. To improve data quality, it is important to establish clear and consistent standards for data storage and formatting, and to regularly verify and update the data to ensure accuracy.
What is the difference between Data Quality Management vs “Master” Data Management (MDM)
Data quality management tools are software solutions that are designed to help organizations ensure the quality of their data. These tools typically provide features for verifying and cleaning data, as well as for establishing and enforcing standards for data storage and formatting.
Master data management (MDM) software, on the other hand, is specifically designed to help organizations manage the core data elements that are critical to the organization’s operations in a centralized manner. MDM software provides features for storing, organizing, and maintaining the accuracy and consistency of master data. You might say that an MDM initiative incorporates all of the goals of a data quality initiative, in addition to handling centralized data governance as well.
Why is Data Quality important?
Almost all successful enterprise companies are now driven by data. Data quality, stewardship, and governance are crucial for making informed business decisions and leveraging the power of AI and machine learning. Data must be accurate, complete, and easily accessible to decision-makers within the company. Data agility is necessary for businesses to remain competitive, and individuals working in data quality, stewardship, and governance programs are responsible for ensuring data correctness and completeness. The scope of these initiatives may vary, but most companies incorporate data management into their strategic plans.
Does Firstlogic provide a Data Quality Software solution?
General purpose database tools are usually inadequate for the heavy-lifting of data quality management. Companies that are serious about clean data and establishing a reliable customer data platform rely on specialized data quality tools from data experts like Firstlogic. Our customers use the Firstlogic Data Quality IQ Suite to update, standardize, and combine data using batch jobs or the optional enterprise developer SDK.
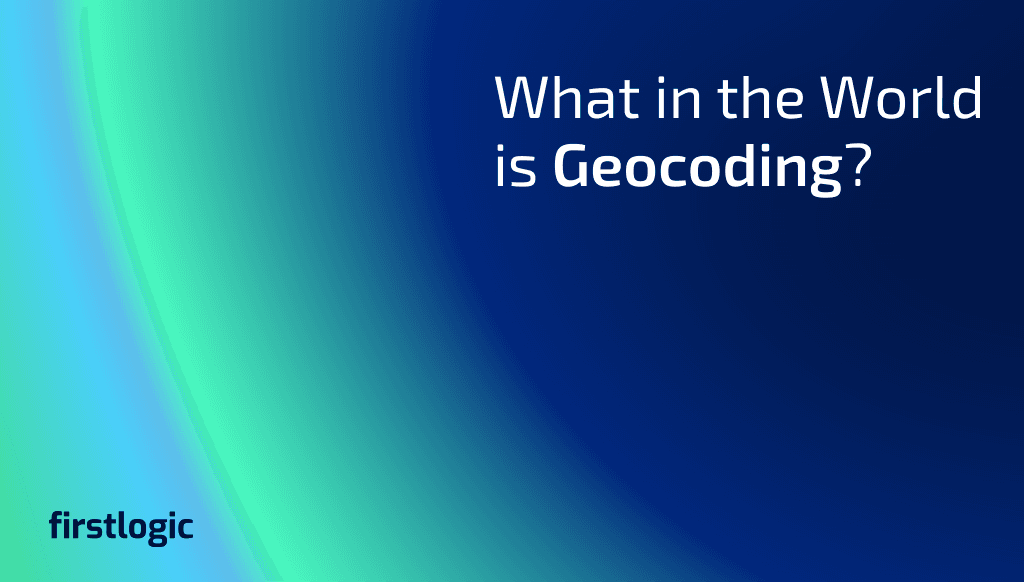
What in the World is Geocoding?
Geocoding connects a postal address to a physical point on the planet. Adding this information to a corporate database enables marketers to do many things they cannot do with addresses alone. Today we will learn how geocoding works, the levels of accuracy involved, and how organizations can take advantage of this data in mailed and […]
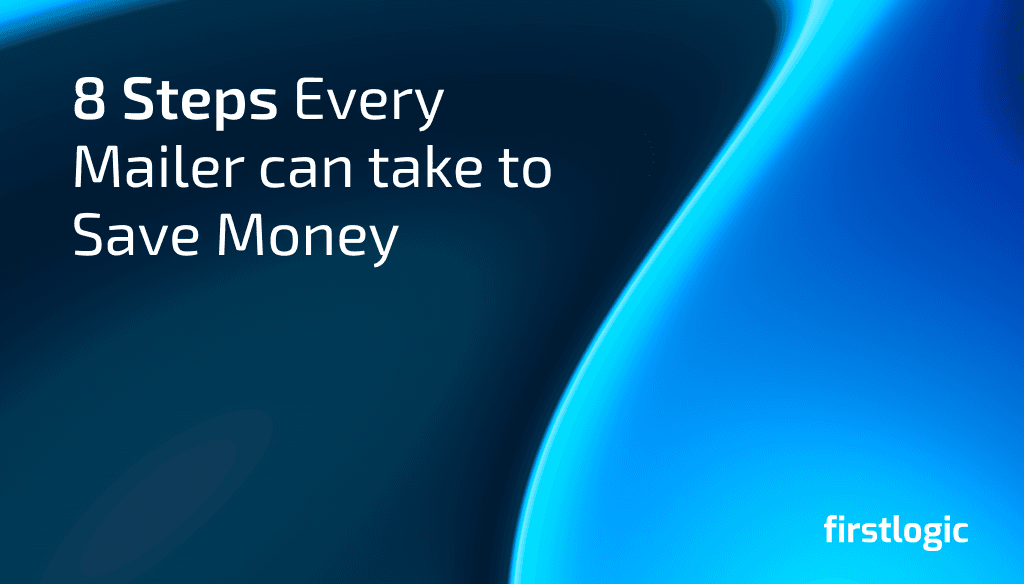
8 Steps Every Mailer Can Take to Save Money
The new USPS rate-setting authority allows the Postal Service to adjust postage rates more aggressively than in the past. Analysts are advising mailers to prepare for twice-per-year rate increases for the next few years. To avoid spending more than necessary, mailers must take every step possible to decrease the impact of postage rate hikes. Some […]