What is a Data Lake?
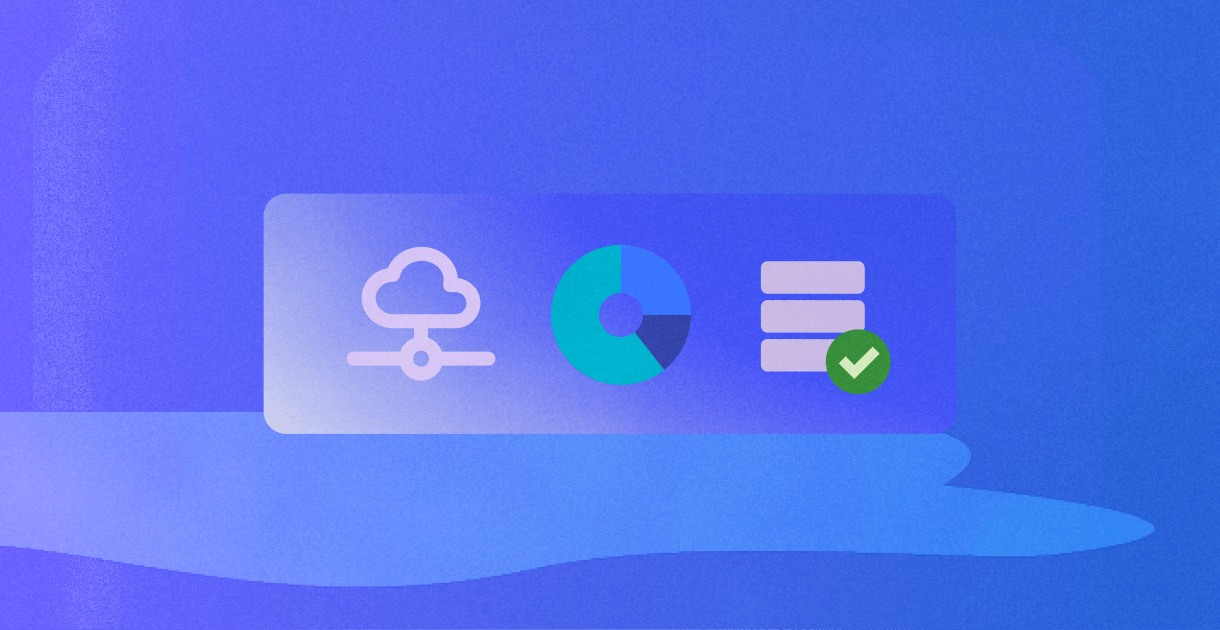
Learn more about this low cost and flexible storage technology and how it can benefit from data quality tools.
Businesses are constantly seeking ways to extract actionable insights from the vast amounts of data they generate daily. Central to this quest is the concept of a “data lake”. A data lake is a large, scalable storage repository that holds raw data in its native format until it’s needed. This storage environment is low cost and can store both structured and unstructured data, ranging from database dumps to social media feeds, without requiring a predefined schema. When businesses need to analyze their data, they can pull it directly from the data lake and transform it to fit their specific use case.
One advantage of a data lake is its ability to store data at any scale. Given the exponential growth of data, it’s crucial for businesses to have a scalable repository that can handle petabytes of raw data. Since a data lake does not require a predefined schema, businesses can store data as-is, ensuring they capture all data points necessary for future analysis.
Data lakes are not without their complexities, however. In the absence of a predefined schema, ensuring data quality can be a significant challenge. Data quality tools and strategies are, therefore, essential for maintaining the integrity and reliability of the data stored within a data lake.
What Are the Differences Between a Data Warehouse and a Data Lake?
Data warehouses and data lakes both store large amounts of data, but they differ in their approach to data storage and processing. Data warehouses store structured data that has been processed and transformed for analysis. They typically use a defined schema, so companies must structure data in a specific way before they can store it. Data warehouses are optimized for querying and analysis, and they are typically used for business intelligence and reporting applications.
Conversely, data lakes accommodate a wider spectrum of data types, including unstructured and semi-structured data. They can store raw data with no need for a predefined schema, providing a flexible and scalable storage solution. As a result, data lakes offer a more holistic view of an organization’s data assets, allowing data scientists and analysts to extract valuable insights from a myriad of data sources.
Data Lakes | Data Warehouses | |
Type of Data | Stores both structured and unstructured data | Primarily stores structured data |
Schema | Schema-on-read, flexible | Schema-on-write, predefined |
Storage Cost | Relatively low | Relatively high |
Users | Data Scientists, Machine Learning Engineers | Business Analysts, Data Analysts |
Both data warehouses and data lakes play crucial roles in data management strategies. The choice between the two depends on the specific needs and goals of a business. Large enterprises often use both, using data lakes for raw data storage and exploration, and data warehouses for structured data analysis and reporting.
Why Are Enterprise Companies Turning to Data Lakes?
In today’s data-driven business landscape, enterprise companies are increasingly turning to data lakes as a solution to manage vast amounts of data. There are several compelling reasons for this shift.
Data Variety and Volume: Traditional database systems often struggle with handling data volume and variety. Data lakes do not need a predefined schema to store data, making them highly flexible in accommodating diverse data types.
Cost-Effective: Data lakes are more cost-effective for storing large volumes of raw data. This cost-effectiveness results from the use of commodity hardware for storage and the ability to scale storage up or down as needed, providing significant cost savings over traditional data warehouses.
Machine Learning Capable: Data lakes allow for advanced analytics like machine learning and AI to be performed directly on the raw data, providing enterprises with deep, actionable insights.
Scalability: Data lakes are highly scalable, allowing them to grow with the needs of the business. Unlike traditional databases that can become overwhelmed as data grows, data lakes can handle the increasing volumes of data generated by modern businesses.
Data Quality Tools Integration: Data lakes seamlessly integrate with data quality tools. These tools can help clean and standardize data entering the lake, ensuring it is fit for use when it is eventually consumed. This integration can help support the accuracy, completeness, and reliability of the data stored in the lake.
The Advantages of Storing Both Structured and Unstructured Data
First, the versatility that data lakes offer in terms of data storage is unparalleled. Unlike traditional databases that necessitate the need for structured data, data lakes can accommodate all types of data, including structured data, such as relational data, and unstructured data, such as email, images, and videos.
The capacity to store both structured and unstructured data gives businesses the freedom to accumulate and analyze diverse data types, enabling a more comprehensive understanding of business operations and customer behavior.
Second, the ability to store unstructured data, which makes up a significant proportion of all data generated, opens vast possibilities for data exploration and analysis. With traditional databases, important insights might be overlooked because of the limitations in handling unstructured data. Data lakes facilitate deeper insights and drive better business decisions.
Data lakes offer a cost-effective solution for big data storage. The scalability of data lakes allows for the storage of large volumes of data at a lower cost than traditional databases. Businesses can store unlimited amounts of data and scale up or down according to their requirements, thus optimizing storage costs.
Integrating Data Quality Tools with Data Lakes
Given the unstructured nature of data lakes, integrating data quality tools is a critical step for businesses looking to glean valuable insights from their data. These tools help guarantee the data is correct, complete, consistent, and reliable–ensuring trustworthy data analysis.
Data quality tools integrate with data lakes through a process known as “data ingestion”. During this process, applications read data from various source systems and bring it into the data lake. As part of the ingestion process, data quality tools can validate the data, find duplicates, and perform necessary transformations, ensuring the data is fit for the purpose. SAP’s Data Quality Management products help companies ensure their data lakes are populated with high-quality information.
Data quality tools make data governance in data lakes possible by enforcing data consistency, security, and privacy regulations. This ensures the data stored in data lakes complies with industry standards and regulatory requirements, reducing the risk of data breaches and non-compliance penalties.
Data Governance in Data Lakes
Ensuring data governance in a data lake is a complex process that involves a series of activities and tools. In this data-driven era, enterprise companies are increasingly reliant on data lakes for their storage capabilities and their flexibility in schema-less data ingestion. However, the ease of ingesting raw, unstructured data into a data lake often comes with the challenge of maintaining data quality and governance. A robust data governance strategy is crucial to maximize the utility and integrity of the data within your data lake.
Data governance in a data lake involves setting up policies, procedures, and frameworks to manage data quality, privacy, security, and compliance. These governance measures not only ensure the accuracy, consistency, and reliability of the data, but also ensure compliance with regulations and standards.
Maintaining data quality is not a one-time task, but requires ongoing monitoring and management. Therefore, data quality tools should provide continuous data quality checks and alerts to potential issues.
Data Quality: By setting up proper rules and procedures, data governance helps in maintaining the quality of data stored in the data lake.
Data Privacy and Security: Data governance measures ensure the privacy and security of data, protecting it from unauthorized access and breaches.
Compliance: Compliance with regulations and standards is crucial in any data environment. Data governance helps in meeting these compliance requirements.
For more information about how the SAP Data Services Platform enables enterprises to leverage the power of data lakes, contact us here at Firstlogic.