What is a Data Mesh?
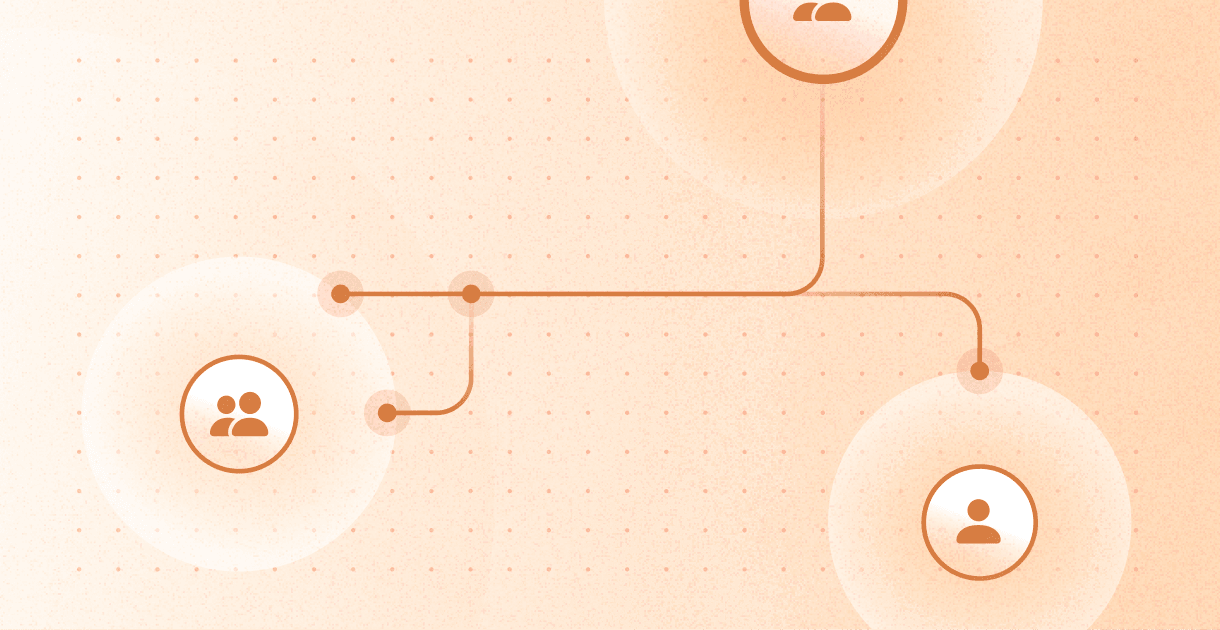
A new term, the data mesh, describes an innovative method for data management.
A new term, the data mesh, describes an innovative method for data management. Differentiating distinctly from the common centralized data architectures, the data mesh concept realigns the core methods by which organizations interpret, manage, and leverage their data.
A data mesh is a decentralized, domain-oriented, and product-centric data architecture that promotes the democratization of data and places a strong emphasis on data ownership and governance. Distinct from traditional data management systems, the data mesh challenges the monolithic, centralized nature of traditional data architectures.
While both are associated with enabling better data use throughout large organizations, a data mesh is an entirely different concept from a data fabric. Data fabrics encourage data access via metadata powered by artificial intelligence, but a centralized data team maintains responsibility for data governance. In a data mesh environment, business units, who are presumably most familiar with the data for which they are responsible, assume more of the work to ensure data quality and accessibility is maintained.
The Data Ownership Difference
In a data mesh system, a dispersion of data ownership necessitates a shift in the role of product teams. They are no longer simply consumers of data, but also custodians, possessing both a sense of ownership and a responsibility for the management and governance of their own data products.
With the implementation of a data mesh, product teams’ duties expand, evoking an integral role in data management. Here are a few critical areas where their responsibility lies:
Data quality: Teams bear the responsibility for the constant upkeep of data, ensuring its accuracy, consistency, and completeness.
Metadata management: Product teams are entrusted with metadata management, which affects data integration and consolidation.
Security and privacy: Product teams under a data mesh are charged with guaranteeing the security and privacy of their respective data sets.
Standardized data formats: A consistent data interface is essential in a mesh. Responsibility falls to the teams to generate and maintain coherent data structures and schemas.
Apart from assuming these responsibilities, product teams also facilitate the discoverability of their information, making sure the data is accessible to other cross-functional teams. This approach bolsters organizational transparency and increases opportunities for data synergy, consequently uplifting the overall value of the organization’s data assets.
Infrastructure Implications
Another critical distinction of the data mesh approach lies in infrastructure implementation. While traditional architectures often employ unified storage and computation systems, a data mesh favors a dispersed approach. Rather than having all data stored and processed in one location, it is distributed across a self-serve data infrastructure, enabling faster access, and eliminating potential bottlenecks.
A data mesh aims to integrate the various domains of an organization into a unified whole, promoting data access equality, sharing, and cohesiveness.
Is a Data Mesh Right for Your Organization?
Swift data processing and access to relevant, valuable data have become integral to company objectives for optimizing operations and driving business growth. The data mesh is an innovative alternative, aiming to tackle challenges of older data platforms that struggle to cope with ever-expanding data volumes.
A data mesh, initially conceptualized by Zhamak Dehghani in his book “Data Mesh: Delivering Data-Driven Value at Scale”, is a stark departure from the traditional central data warehouse or data lake model. The idea shifts away from a top-down, centrally controlled data arrangement.
Organizations should consider several factors when considering the suitability of a data mesh:
Scale of data operations: A data mesh can shine in scenarios where organizations must manage large-scale data operations spread across multiple geographies and functions. It encourages a more collaborative, distributed approach that effectively uses the local knowledge of each autonomous team.
Expertise and resources: Deploying a data mesh requires adequate ability, commitment, and resources to build cross-functional teams capable of managing their own domain-oriented datasets. This includes not just understanding the data itself but mastering the technology supporting its processing and storage.
Organizational culture: Ultimately, the success of a data mesh relies on how well an organization can adapt to a culture of distributed data ownership. Organizations with traditions that support innovation, autonomy, and accountability are more likely to experience a successful data mesh implementation.
Transitioning to a Data Mesh
Experts recommend organizations pilot a data mesh project with a single business case. Identify situations where product teams are willing to take on data responsibility. The central data governance team should define the self-service routines for the business unit and build the data product before turning it over to the product team. Establish a road map for implementation and include data governance at each step. The data governance team should be accessible during the pilot to advise, assist, and monitor the progress of the business unit administering the pilot project.
It is crucial for organizations aiming to adopt a data mesh to understand their current data platforms, be they data lakes, data warehouses, or a blend of the two. Before initiating the transition to a data mesh, the development of a comprehensive plan is paramount. This plan must outline the evolution of their present data platform in synchronization with the growth of the emerging data mesh.
A few integral factors require careful contemplation from these organizations:
The data resources: Identifying the data resources that perform optimally within the context of a data mesh is critical.
The core assets: Determining the assets that need to be retained within the initial data platform is fundamental.
The asset location: Evaluate the necessity for assets to be moved, or if maintaining them on the current platform while still engaging in the data mesh is preferable.
Ensuring Data Accuracy: Leveraging Data Quality Tools in a Data Mesh
How an organization integrates and uses tools within a data mesh architecture makes a difference in the project’s success. Data quality tools play a distinctive role here. These tools help ensure the data feeding into analytics or business intelligence systems is accurate, consistent, and reliable.
In a data mesh, each autonomous team should treat their domain-oriented data set as a product they own, update, and maintain. For these teams to have confidence in the data they manage and share, implementing data quality tools is crucial.
Companies can promote a culture of data quality among product teams. They may achieve this by offering education and training, inspiring the best practices and standards of data quality, supporting data quality improvements and achievements, and endorsing collaboration and feedback.
Data quality tools can identify issues such as duplicates, inconsistencies, or missing values, ensuring each domain’s data is clean, well-structured, and ready for analysis. They ensure the integrity, accuracy, and consistency of data across the distributed data ecosystem. Unlike traditional architectures where data quality checks are centralized, in a data mesh, these health checks are distributed and woven into the mesh itself.
Data validation: Data quality tools confirm the data at its point of origin, which ensures accuracy right at the source.
Data profiling: These tools offer insights into the characteristics of the data, promoting further understanding of data structure, relationships, and potential inconsistencies.
Data cleansing: Cleanse data by identifying and rectifying errors or inconsistencies within the data sets.
Data feedback: Several mechanisms, such as data quality dashboards, alerts, workflows, and data quality forums provide ongoing reporting and resolution, creating a continuous feedback loop.
Thinking About a Data Mesh?
The concept of a data mesh stands out for its innovative approach to resolving challenges businesses face in harnessing vast volumes of data. Organizations can benefit from examining the multi-faceted dimensions of a data mesh and the careful considerations required for adoption.
The most striking characteristic of a data mesh environment is its implication for data ownership. It decentralizes data management and assigns specific data domains to cross-functional teams. This shift transforms the typical centralized data ownership model, introducing a widespread sense of accountability for data quality. It disperses the control of data across the organization, fostering a more democratic, inclusive, and effective approach to data asset management.
The adoption of the data mesh model often requires re-imagining an organization’s traditional data infrastructure. The applicability of a data mesh is often determined by several factors pertaining to an organization’s data landscape. Considerations include the complexity and scale of data, the capabilities of product teams, and the willingness to adopt innovative technological changes.
Transitioning to a data mesh architecture is a strategic shift that encompasses data, technology, and people. Notably, it requires a gradual transition enabled by pilot projects, financial investments, training, administration, and skilled technical support when needed.
The conceptual shift towards a data mesh architecture brings with it a host of opportunities and challenges. Organizations should be deliberate in their approach to securing a transition that benefits all teams and promotes superior data quality. The implications of a data mesh approach extend far beyond merely technical changes. The architecture paves the way for a more inclusive and efficient data management paradigm that fosters agility, innovation, and greater exploitation of data assets.
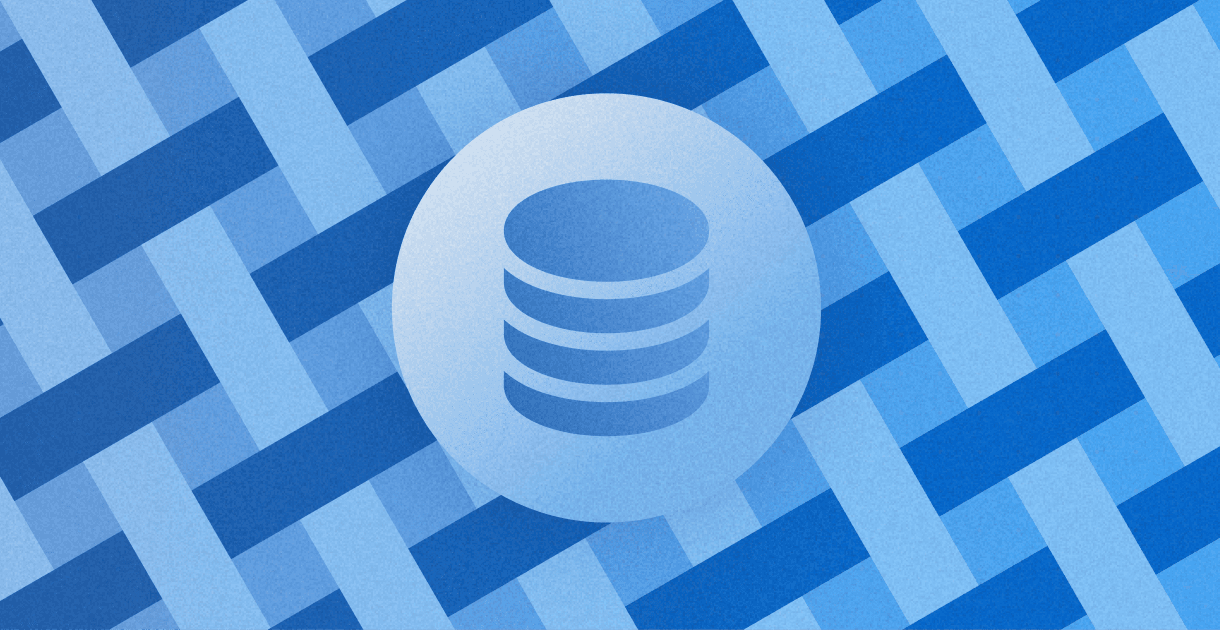
What is a Data Fabric? Making Sense of the Trend
How data quality tools fit into this novel data management architecture.
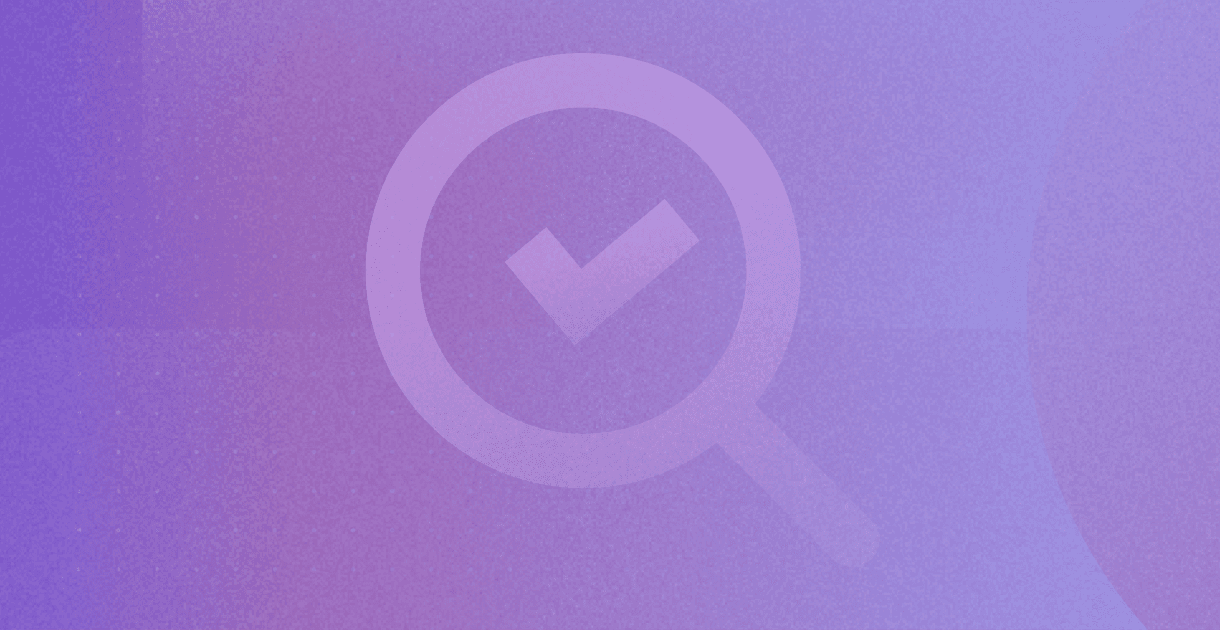
What is Data Profiling?
Data profiling is analyzing and examining data to gather insights and understand the quality, structure, and content of the data. It involves examining various attributes of the data, such as data types, patterns, relationships, and values. With data profiling, organizations can gain a comprehensive understanding of their data, which is crucial for ensuring data quality […]