You Can’t be Efficient if Your Data is a Mess
Data comes to an organization from many sources. Much of the data is produced by systems with different guidelines, formats, and standards. Are you handling it properly?
To compete on a global scale and deliver the best possible experience to customers, many companies are concentrating their efforts on advances in efficiency and automation. The ability to make progress in these areas depends on the data available to your organization. This can be an issue if your company has not taken steps to ensure your data is accurate, standardized, and consolidated.
Data comes to an organization from many sources. Besides the unconnected data silos your company creates for itself, other data is customer-provided, comes from vendors or partners, from third-party sources, and more. Much of the data is produced by systems with different guidelines, formats, and standards. No wonder companies struggle with data as they attempt to create reports and analytics used to make decisions about efficiency and automation.
If your organization has merged or has acquired another company, the data quality task multiplies.
Let’s look at some common data quality challenges that can affect your company’s ability to achieve efficiency objectives.
Territory Boundaries
Companies seeking to gain efficiencies in the field want to allocate sales or service resources in a manner that minimizes travel time and maximizes productivity. To do this you must know where your customers are located and how many exist in a geographical area.
Accurate geocoding is essential, but before assigning latitude and longitude coordinates to a data record, you must be sure each address exists and is complete and correct. Otherwise, decisions based on the distribution of customers will be flawed, resulting in zones that undermine territory optimization efforts, rather than strengthen them.
Because of their street addresses, two customers may appear to be near each other. A simple error in street directionals, however, such as listing a location on NW Beltway instead of NE Beltway, can be misleading. A single wayward character in an address could turn an expected walk to the business next door into a thirty-minute drive.
Retail Site Location
Choosing the right location for a retail outlet can be the difference between success and ultimate failure. Selecting a site near large clusters of customers is a good strategy, unless your customer database is out of date. Neglecting to remove duplicate customers or people who have moved, died, or no longer meet your buyer profile criteria will skew the data and could lead to an expensive mistake.
Computed Shipping Costs
Customers buying products online want to know the shipping cost, and how long it will take for their orders to arrive. Automatically providing such information while a customer is completing their online order is ideal, but you immediately set expectations customers will demand you fulfill.
Delivery delays caused by insufficient address data trigger a poor customer experience, complaints, and negative reviews. Late packages spur angry inquiries for your customer service representatives to handle. Inaccurate shipping cost calculations that underprice shipping charges erode your profit margins.
Verifying the accuracy of a customer’s address before quoting shipping charges requires a quick and efficient address quality workflow.
Data Consolidation Issues
Strategies associated with areas such as customer retention and upselling require a complete understanding of your company’s relationship with individual customers. This usually involves analyzing data from multiple sources to collect information in areas such as purchase history, loyalty, or credit rating. If your data is poorly formatted, this data consolidation effort will be difficult.
Suppose one database lists customer names in a single field. Another database logs customer names in separate fields for first and last names. A third source may rely on an account username and a shipping address. How will you determine which data records to combine?
Data quality software that includes advanced matching and consolidation logic will make this task manageable, resulting in an accurate representation of each customer relationship.
Solutions from Firstlogic
Firstlogic provides data quality tools that can help companies organize and cleanse their data for maximum operational efficiency. Firstlogic DataRight IQ® helps to parse and standardize data from multiple sources while Match/Consolidate® uses advanced matching algorithms to find data relationships for consolidation. In addition, Firstlogic ACE® will correct bad addresses while Geocode IQ™ will assign the latitude and longitude for precise location analysis and reporting. And for large enterprise service providers, the SAP® Data Services platform delivers the entire suite of data quality functions in a single enterprise platform. Efficiency and automation are probably on your to-do list in the coming months. Use software and services from Firstlogic to make sure your efforts are based on high quality data.
Geospatial Marketing – How to Pinpoint the Right Customers
Postal address information is important. Standardized and corrected postal addresses are essential for accurate mail delivery, targeted marketing, and other activities a business undertakes to run its operations. Postal addresses do not, however, always identify a precise physical location on Earth. When precise location is important, you must add geographic latitude and longitude coordinates to […]
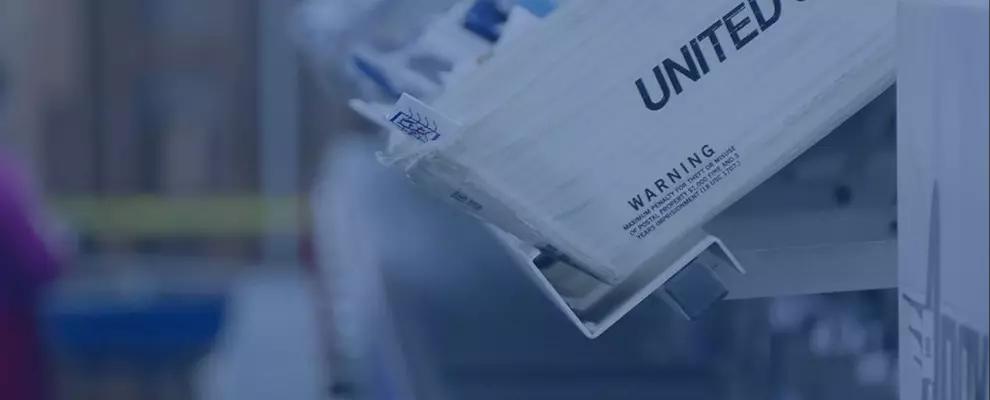
USPS® Address Verification | Free Options and Best Tools
USPS Address Verification validates and standardizes mailing addresses to ensure accurate and timely mail delivery. The United States Postal Service (USPS) offers an address verification service that confirms address information and corrects any errors or inconsistencies. Address verification involves comparing address information to the USPS database and standardizing it to conform to USPS formatting rules. […]