A Business Guide to Data Accuracy
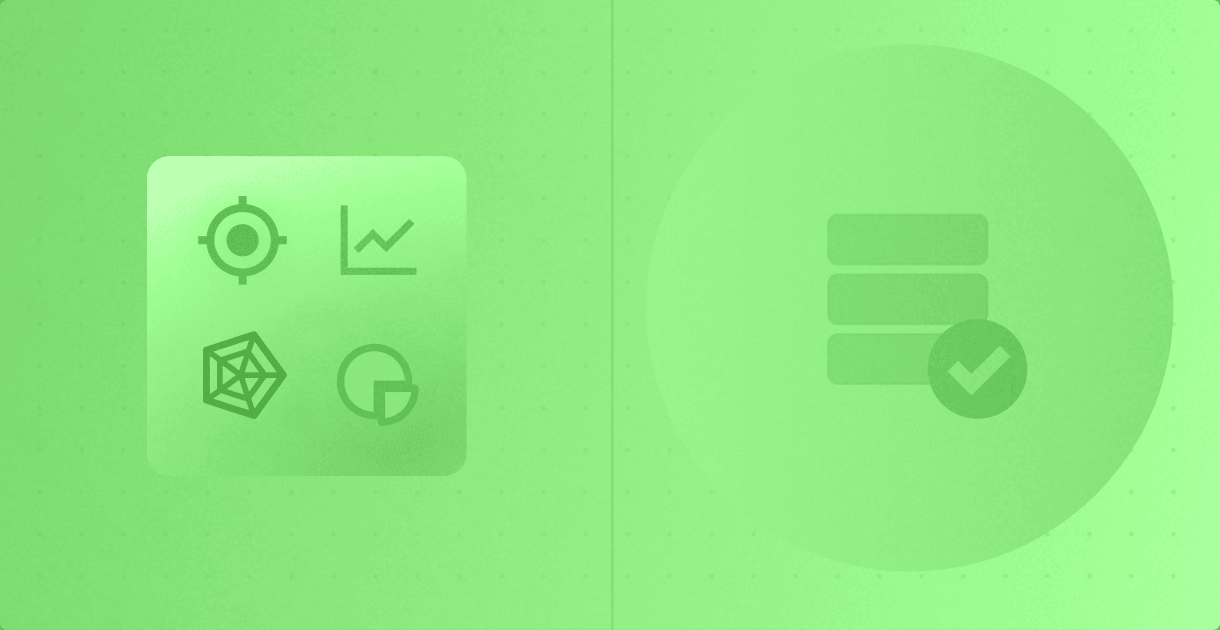
Knowing your business becomes a guessing game if the correctness of your data can’t be trusted.
Everything about your business becomes a guessing game if the correctness of your data can’t be trusted. Precise data isn’t just a desire; it’s a need.
According to studies by Gartner, poor data quality costs businesses an average of $12.9 million every year. Clearly, ensuring data accuracy is a strategic effort that can affect any company’s success. Accurate data is a critical foundation of informed decision-making. Maintaining it should be a top priority in all organizations. Data accuracy can drive strategic initiatives, streamline operations, and reduce the risk of costly errors. Conversely, data oversights can lead to expensive mistakes and missed opportunities, negatively affecting the bottom line. Mastering the strategies and techniques required to obtain and manage correct data is of paramount importance.
- Organizations must address three key areas of corporate-wide data quality:
Data Entry: Accurate data starts at its source. Training employees on the importance of precise data entry is vital. This includes areas such as correct spelling, duplicate data, and consistently formatting information across all platforms. - Data Validation: Regularly checking and confirming the accuracy of data is essential. Automated checking mechanisms can confirm this accuracy, identifying and flagging suspicious entries for manual review.
- Data Quality Control: Implementing rigorous quality control measures helps ensure the continued accuracy of data. This includes regular audits, reviews, and updating data as necessary.
Considering these challenges, having a reliable partner in maintaining data accuracy is crucial. The Firstlogic Data Quality IQ Suite® is such a partner, providing comprehensive data management solutions that encompass these three fundamental aspects of data accuracy.
The Importance of Maintaining Accurate Business Data
Maintaining data accuracy is important for the success of a business for several reasons. Good data is crucial for making informed business decisions. When data is correct, companies can confidently analyze trends, identify patterns, and gain insights into customer behavior. This allows businesses to make strategic decisions based on reliable information, leading to better outcomes and increased profitability.
Errorless data is essential for effective customer relationship management (CRM). When customer data is correct, businesses can personalize their marketing efforts, tailor their products or services to specific customer needs, and provide exceptional customer experiences. Inaccurate data can lead to miscommunication, missed opportunities, and a negative impact on customer satisfaction.
Regulatory compliance is another business area that depends on factual data. Many industries have strict regulations regarding data privacy and security, such as the General Data Protection Regulation (GDPR) in the European Union. With California leading the way, individual states in the US are ramping up their data collection and use laws. Maintaining solid data ensures businesses can follow these regulations, avoid legal issues, and protect their reputation.
Efficient company operations and cost savings are only achievable with correct data. Inaccurate data can cause organizations to waste resources, such as sending marketing materials to incorrect addresses or contacting customers who have opted out. By maintaining data accuracy, businesses can decrease errors, reduce operational costs, and improve overall efficiency.
Potential Consequences of Inaccurate Business Data
Data inaccuracies in a business can lead to various negative consequences. One potential outcome is incorrect business decisions. When data is inaccurate, it can misrepresent the true situation, leading decision-makers to make choices based on faulty information. This can cause poor strategic planning, ineffective resource allocation, and missed opportunities for growth and profitability.
Customer satisfaction declines when companies operate with inadequate data. Inaccurate customer data can lead to errors in communication, such as sending marketing materials to the wrong addresses or including off-putting personalized references in customer communications. This can damage the relationship between the business and its customers, leading to dissatisfaction, loss of trust, and ultimately, customer churn.
Data errors can have financial implications for a business. For example, inaccurate financial data can lead to errors in budgeting, forecasting, and financial reporting. These errors can cause financial losses, regulatory non-compliance, and even legal consequences. Inaccurate data can also impact inventory management, leading to overstocking or under-stocking of products, which can tie up capital or result in lost sales.
Marketing and sales efforts suffer when hindered by inaccurate data. When customer data is wrong, targeted marketing campaigns may not reach the intended audience, resulting in wasted resources and missed opportunities to generate leads and drive sales. Poor data quality also leads to ineffective sales forecasting, making it difficult to predict customer demand and allocate resources accordingly.
Bad data can negatively impact the overall efficiency and productivity of a business. Erroneous data can lead to time wasted on manual data correction, troubleshooting, and rework. It can also result in delays in decision-making processes, as stakeholders spend time verifying and validating data before making informed choices. These inefficiencies can hinder business operations, slow down growth, and increase operational costs.
Practical Tips and Strategies for Data Accuracy
To protect data accuracy in an organization, companies can implement several practical tips and strategies. Clear data entry guidelines and standards are crucial. This includes defining data fields, formats, and the required information for each data entry task. By providing explicit instructions, employees can enter data consistently and accurately, reducing the chances of errors and inconsistencies.
Implementing data validation processes is another effective strategy. This involves using automated tools or software to validate data entries against predefined rules or criteria. For example, validating email addresses to make sure they are in the correct format or checking numerical fields for valid ranges. By implementing data validation, organizations can catch errors quickly and prevent inaccurate data from spreading throughout their systems.
Regular data quality control checks are also essential. This involves conducting periodic audits of the data to find any inconsistencies, errors, or duplicates. By regularly reviewing and cleaning the data, organizations can uphold its accuracy and integrity. Companies can review data manually or use data quality software that can automate the process and provide detailed reports on data quality issues.
Training and educating employees on the importance of data accuracy is another crucial step. By providing training sessions or workshops on data best practices, employees can learn how to enter and nurture data. Additionally, organizations should emphasize the importance of data accuracy and its impact on business success. This can help create a culture of data accuracy and encourage employees to prioritize accuracy in their daily tasks.
An established data governance framework can contribute to data accuracy. This involves assigning roles and responsibilities for data management, creating data policies and procedures, and implementing data quality metrics and monitoring. By having a structured approach to data governance, organizations can consistently control and monitor data throughout the organization.
Data Best Practices
Data Entry
To ensure data accuracy as it enters the organization, it is important to establish clear guidelines and standards for data entry. This includes training employees on proper data entry techniques, such as using consistent formats, standard abbreviations, and double-checking entries for errors. Implementing data validation rules can also help catch and correct errors during the data entry process.
Some common data entry issues include:
- Transposition – Data entry operators inadvertently transpose characters or digits, leading to errors that downstream quality control measures may or may not catch. Tools like Firstlogic’s Address IQ® will discover and correct an error in a house number or street name. However, such tools may not flag two characters transposed in a phone number or email address.
- Misinterpretation – This error happens when data entry operators must make a guess. Is that a one or an “I”? An “S” or a five? A capital “C” or lower-case? With an emphasis on speed, data entry operators have little time to evaluate the material in front of them before moving on to the next document. Arbitrary decisions made at data entry time can make a difference later.
- Data formatting – This can be especially tricky when data entry involves multi-national entities. A date expressed as 3/9/23 represents March 9th in the US, but September 3rd in other countries. Other differences occur in currency and surnames. In some parts of the US, directionals like NW or SE precede the street name. In other locations, they follow the name. Address quality software like Address IQ will catch and correct most postal address formatting errors.
- Misplaced data – Software that compares entered data against business rules can catch instances where the right information is recorded in the wrong column. Accidentally switching data between two free-form data columns, however, will be tougher to discover.
With APIs that connect their data entry software with the Firstlogic Data Quality Suite, organizations can correct many common errors at the best possible time – while data is being entered. With adequate precautions, bad data never becomes embedded in databases across the enterprise.
Data Validation
Data validation is a process that verifies data is accurate, complete, and consistent before the data is used or processed further. One best practice for data validation is using automated validation tools or software. This software can perform checks on data integrity, format, and completeness. These tools can help identify and flag any inconsistencies or errors in the data, allowing for timely corrections and ensuring data accuracy.
Companies validate data by comparing data inputs against a data model. This comparison may occur in various steps as data moves about the enterprise, including ETL (extract, translate, and load) or data warehousing.
Some data validation steps most often deployed by organizations include:
- Data type – A data type validation can catch errors, such as alpha characters in a numeric field or invalid special characters.
- Length – Some fields have a limit on the minimum or maximum number of characters. Passwords, for instance, are often required to be at least eight characters. Social Security or tax ID numbers are always exactly nine digits.
- Look up – This validation measure checks data against a list of acceptable values. Validating US state abbreviations, for example, prevents an organization from maintaining data corresponding to a non-existent state. The lookup table includes only the valid state abbreviations for 50 US states plus any acceptable US territories or possessions.
- Uniqueness – Sometimes, a value can occur only once in a database. Email addresses, Social Security numbers, or product serial numbers are examples.
Tools like Data Quality IQ®, part of Firstlogic’s Data Quality Suite, handle the data validation chores for companies. Use software to automate enterprise-wide data validation. Companies can safely distribute validated data throughout the organization and use it for any purpose the company needs.
Quality Control
Quality control ensures that data meets the desired level of quality. This involves regularly monitoring and reviewing data for accuracy, completeness, and relevance. One best practice for quality control is to establish data quality metrics and benchmarks, which can serve as a standard for evaluating the accuracy of data. Regular audits and reviews of data can help pinpoint any issues or discrepancies and prompt data specialists to initiate corrective actions.
Another best practice for quality control is to implement data governance policies and procedures. This includes assigning roles and responsibilities for data management, establishing data quality standards, and implementing data stewardship programs. By implementing clear guidelines and accountability measures, organizations can be certain data accuracy is a priority and all employees are aware of their roles in maintaining data quality.
Regular data cleansing and maintenance are also crucial for data accuracy. This involves identifying and removing duplicate, outdated, or irrelevant data. By regularly cleaning and updating the data, organizations work with factual and up-to-date information. Data quality tools can streamline the data cleansing process and help maintain data accuracy over time.
Here are a few data quality best practices:
Executive support – Support from the C-suite is critical to the success of corporate-wide data quality initiatives. Without such endorsements, managers and employees may view data quality as an imposition and resist complying with data quality standards.
- Training – Make it a priority to include training for employees to help them understand the impact of data quality on the health of the organization and their own career advancement.
- Guidelines – Institute a set of roles, standards, procedures, and metrics with which the company can govern a data quality program. The guidelines may be specific to an organization’s unique processes, use cases, and structure.
- Stewards – Someone with knowledge of major data sets should certify the data meets the data quality standards. Data stewards may be assigned by departments or work as part of the IT organization.
- Integration – Eliminate duplicate or unreliable data sources. Create connections between applications and a single source of truth within the corporation’s data structure.
- Audits – Establish methods to check the data periodically to confirm data quality measures are still being followed and generating reliable results.
Effective quality control often requires a workplace culture adjustment. Participation from everyone throughout the organization is critical. Rogue non-conformist employees can derail enterprise initiatives for a data quality program. Ignored rules allow flawed data to infect the databases. Unreliable data lowers employee confidence in the data and the procedures developed to protect and deploy it.
Best practices for data entry, validation, and quality control include establishing clear guidelines for data entry, using automated validation tools, implementing data governance policies, conducting regular data audits, and performing data cleansing and maintenance. By following these practices and using data quality software, businesses can enhance data accuracy and improve overall business success.
For more information about data management concepts, techniques, and the impact of data quality, see these articles from Firstlogic Insights:
- What is Data Quality? Definition and Frequently Asked Questions
- Data Quality, Data Stewardship, and Data Governance – How Are They Related?
- Data Quality and the Customer Experience
Firstlogic’s Data Quality IQ Suite
The Firstlogic Data Quality IQ Suite® is a comprehensive software solution designed to enhance data accuracy for enterprise customers. It offers a wide range of features and capabilities that help organizations protect the precision and reliability of their data.
One key feature of the Firstlogic Data Quality IQ Suite is its data cleansing functionality. This feature allows users to spot and correct errors, inconsistencies, and inaccuracies in their data. By automatically detecting and resolving issues such as misspellings, duplicate records, and formatting errors, the suite helps improve the overall accuracy of the data.
The Firstlogic Data Quality IQ Suite also offers data enrichment functionality. This feature allows users to enhance their data by adding missing information or enriching existing data with more details. By filling in gaps and enriching the data, organizations can improve its accuracy and completeness.
Overall, the Firstlogic Data Quality IQ Suite is a powerful tool for enhancing data accuracy in enterprise environments. Its comprehensive set of features and capabilities enables organizations to cleanse, substantiate, enrich, and monitor their data, ensuring that it remains complete and reliable.
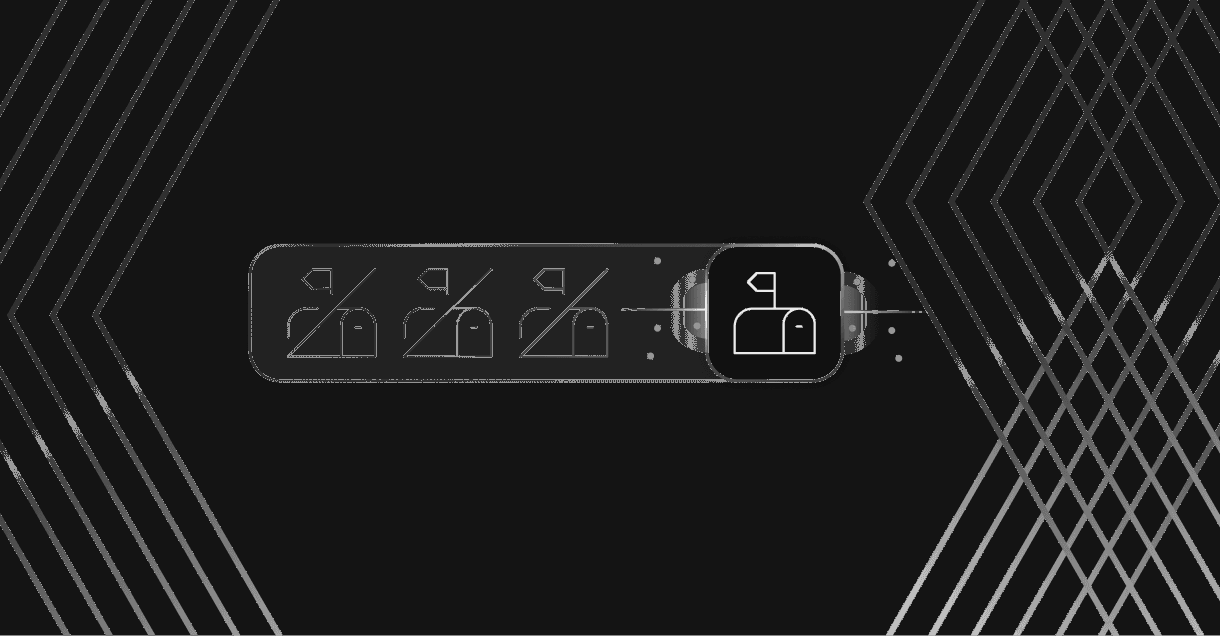
Making the Case for NCOA
NCOA stands for National Change of Address, a service provided by the United States Postal Service (USPS) that allows companies to update their customer databases with the latest address information. This service is crucial for enterprise companies as it helps them maintain accurate and up-to-date customer data. By using NCOA, companies can ensure their mail, […]
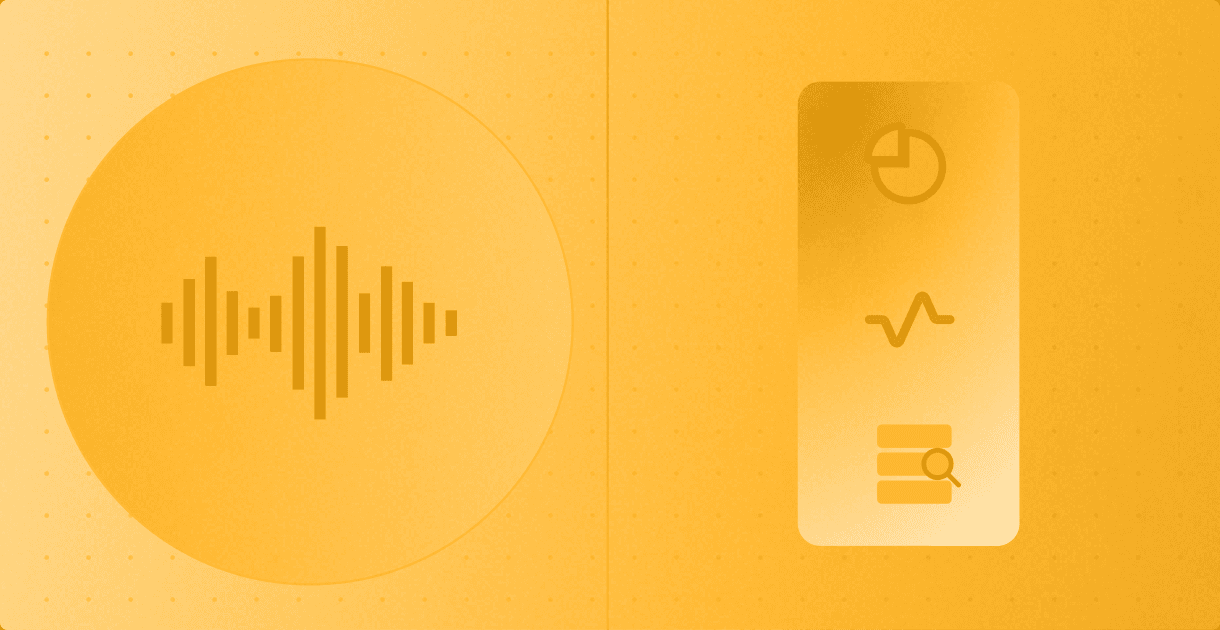
A Business Guide to Fuzzy Matching
Let’s look at two simple data records one might find in any company’s customer database: RECORD 1: John Smith Jr, 123 Washingtn St SW, Atlanta, GA, 30315 RECORD 2: Jonathan K Smith Jr, 123 SW Washington St, Atlanta, GA, 30315 If it were your job to find duplicates manually using common sense, you would likely […]