Data lineage is a pivotal aspect of data management, providing business users with the ability to understand and trace the journey of data from its original source to its final usage. By offering a transparent view of data’s lifecycle, data lineage ensures the reliability and quality of data, which is essential for making informed business decisions.
Definition of Data Lineage
Data lineage refers to the detailed, step-by-step record of the flow of data from its origin through various stages of processing, transformation, and storage, until it reaches its final destination. It encompasses the entire lifecycle of data, providing a map that outlines how data moves through an organization’s systems. This comprehensive view includes where data originates, how it is transformed, and where it is ultimately used, making it an essential component of data governance and data quality frameworks.
To illustrate, consider a retail company that collects sales data from various stores. The data lineage process would track this data from the point of sale systems in each store, through the central data warehouse where it is aggregated, to the business intelligence tools where it is analyzed for trends. Each transformation, such as data cleaning, aggregation, and enrichment, is documented to provide a clear picture of the data’s journey and transformations.
Reasons for Data Lineage
Companies place significant emphasis on data lineage as a crucial component of data quality for several reasons:
Trustworthy Data: Ensuring that data is accurate, consistent, and reliable is paramount. Data lineage provides transparency into the data lifecycle, helping to identify and rectify issues that could compromise data integrity. This trustworthiness is crucial for making accurate business decisions and maintaining customer trust.
Eliminating Data Silos: By mapping out data flows across different systems and departments, data lineage helps break down data silos, promoting a more integrated and accessible data environment. This integration fosters better data sharing and utilization across the organization, enhancing overall efficiency and collaboration.
Compliance: Regulatory requirements often demand thorough documentation and traceability of data. Data lineage supports compliance efforts by providing the necessary audit trails and documentation. Industries such as finance and healthcare, which are heavily regulated, benefit significantly from the ability to trace data origins and transformations to meet regulatory standards.
Simplified Data Operations: Understanding the data journey simplifies data operations, making it easier to manage, transform, and utilize data effectively across various applications and systems. This simplification reduces the complexity of data management tasks and enhances the efficiency of data-related processes.
The Core Parts of Data Lineage
Data lineage consists of several core components:
Data Origins/Sources: This refers to the initial points where data is created or captured. Understanding the origins is crucial for ensuring data authenticity and accuracy. These sources can include databases, data lakes, APIs, and even external data providers.
Data Flows and Transformations: Data flows represent the movement of data between systems and processes. Transformations are the changes data undergoes as it moves through different stages of processing. Documenting these transformations is vital for understanding how data evolves. Examples include data cleaning, filtering, aggregation, and enrichment processes.
Dependencies: Dependencies highlight the relationships and linkages between different data elements and systems. Identifying dependencies is essential for understanding the impact of changes and for effective data management. This includes understanding how changes in one data source or transformation can affect downstream processes and data consumers.
Benefits of Data Lineage
Implementing data lineage offers several advantages:
Proactive Data Governance: Data lineage enables organizations to implement proactive governance measures by providing visibility into data usage and transformations. This helps in setting and enforcing data policies and standards.
Streamlined Troubleshooting: With a clear view of the data journey, it becomes easier to identify and resolve issues. Data lineage aids in pinpointing the root cause of data quality problems, reducing the time and effort required for troubleshooting.
Boosted Collaboration: Data lineage promotes better collaboration among different teams by providing a shared understanding of data flows and transformations. This fosters a more cohesive approach to data management and utilization.
Effective Change Management and Analysis: Understanding data dependencies and transformations helps in assessing the impact of changes. This supports effective change management and ensures that modifications do not adversely affect data quality or operations.
Data Lineage Applications
Data lineage plays a critical role in data observability, providing insights into the health and performance of data systems. By tracing data flows and transformations, organizations can monitor data quality, detect anomalies, and ensure that data processes are functioning as expected. This level of observability is crucial for maintaining high standards of data quality and for supporting data-driven decision-making.
Conclusion
In conclusion, data lineage is an indispensable element of data quality frameworks, providing transparency and traceability throughout the data lifecycle. It enhances data governance, simplifies troubleshooting, fosters collaboration, and supports effective change management. Implementing robust data lineage practices ensures that organizations can maintain trustworthy and high-quality data, which is essential for achieving business objectives.
Firstlogic offers comprehensive on-premise data quality software tailored for enterprise companies, helping them leverage the full potential of data lineage to enhance their data management strategies.
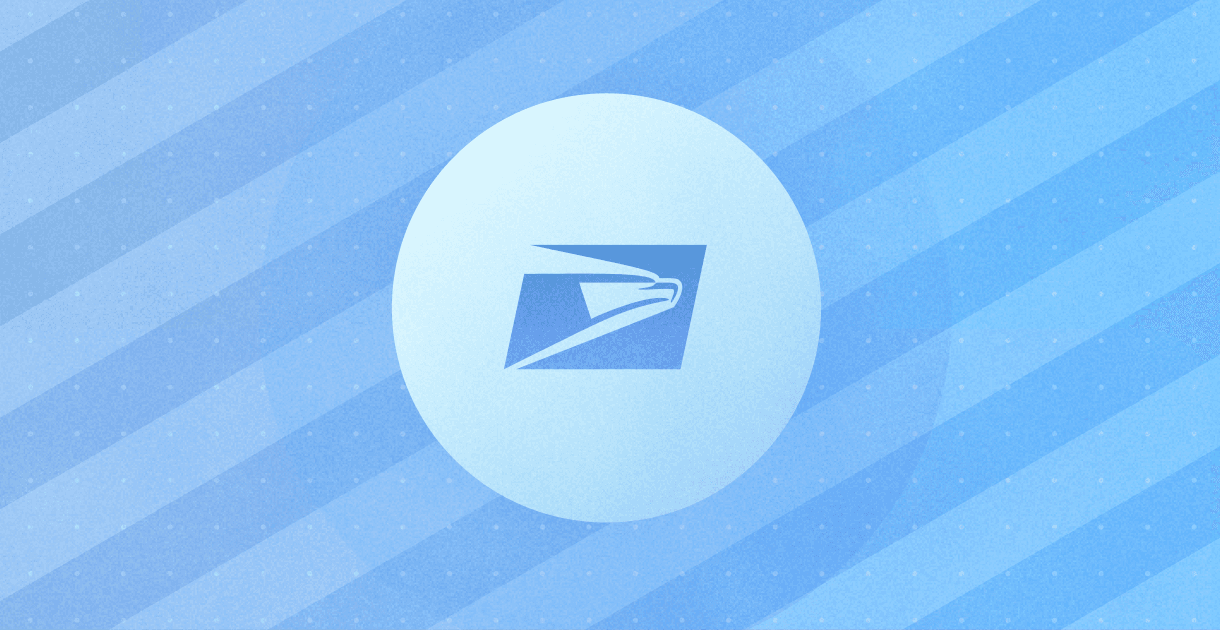
Understanding USPS Returned Mail Codes
USPS returned mail codes are specific codes used by the United States Postal Service (USPS) to categorize and explain the reasons why mail cannot be delivered as addressed.
Data Quality in the Financial Sector
In the financial services sector, inaccurate or outdated data can cause significant issues, affecting countless transactions and interactions. This is why financial data quality management is crucial—poor data impacts many aspects of the business. Consequences of data inaccuracies can be severe, impacting customer experience, revenue, and regulatory compliance. This article highlights the benefits of maintaining […]