Data Quality in the Financial Sector
In the financial services sector, inaccurate or outdated data can cause significant issues, affecting countless transactions and interactions. This is why financial data quality management is crucial—poor data impacts many aspects of the business.
Consequences of data inaccuracies can be severe, impacting customer experience, revenue, and regulatory compliance. This article highlights the benefits of maintaining good financial data quality, the challenges faced by financial service companies, and insights on implementing robust data quality processes.
Benefits of Financial Data Quality
Implementing a comprehensive data quality strategy decreases risk, increases productivity, and drives business growth. Key benefits include:
Enhanced Customer Experience. Accurate data enables personalized and efficient services, improving customer experience. Inaccurate data can lead to miscommunication and dissatisfaction.
Improved Regulatory Compliance. Accurate data helps financial institutions comply with regulations, detect and prevent financial crimes, and avoid penalties and legal issues.
Streamlined Operations. Clean data optimizes processes, reduces errors, and minimizes time spent on manual reconciliation, allowing organizations to focus on core functions.
Informed Decision-Making. Reliable data supports effective decision-making, helping leaders identify growth opportunities and assess risks accurately.
Increased Revenue and Cost Savings. Data inaccuracies can lead to lost revenue and increased costs. Maintaining data quality helps avoid these pitfalls.
Common Data Quality Challenges in Financial Services
Financial institutions face several data quality challenges:
Data Inconsistency. Conflicting information from different sources causes confusion and uncertainty, leading to inaccurate decision-making and increased risks.
Data Fragmentation. Relying on multiple systems leads to fragmented and siloed data, hindering holistic views of customers and transactions.
Incomplete Data. Missing data hinders pattern recognition, risk assessment, and informed decisions, potentially leading to regulatory penalties.
Data Decay. Outdated customer data impacts marketing efforts, customer service, and risk assessments, leading to missed opportunities and increased costs.
The High Cost of Data Inaccuracy in Financial Services
Data quality issues cost financial institutions an average of $15 million per year. Inaccurate data affects customer experience, interactions, and transactions, leading to increased costs, lost revenue, and regulatory penalties.
Role of Data Quality in Preventing Financial Crimes
Maintaining data quality is crucial for detecting and preventing financial crimes:
Accurate Data for Monitoring and Detection. High-quality data enables effective monitoring and detection of suspicious transactions, recognizing patterns, and flagging fraudulent activities.
Compliance with Regulatory Requirements. Accurate data supports compliance with regulations, avoiding fines and reputational damage.
Customer Trust and Reputation. Protecting customers from financial crimes enhances trust and loyalty, improving overall brand image.
Efficient Risk Management. Accurate data allows for better risk analysis, highlighting vulnerabilities and applying necessary controls.
Key Components of an Effective Financial Data Quality Management Strategy
A comprehensive data quality management strategy includes:
- Clearly Define Data Quality Goals: Start by defining data quality goals and aligning them with the financial institution’s business objectives. Identify the specific data quality dimensions that are most critical to the organization, such as accuracy, completeness, consistency, timeliness, and validity.
- Establish Data Quality Standards: Develop clear and measurable data quality standards that define the acceptable levels for each data quality dimension. These standards will serve as benchmarks for evaluating and monitoring the quality of the financial data.
- Data Profiling and Assessment: Conduct a comprehensive data profiling and assessment process to understand the current state of the data. Locate data sources, assess data accuracy, pinpoint data gaps, and evaluate data lineage and data transformation processes.
- Implement Data Validation and Cleansing: Create data validation rules to detect and correct errors, inconsistencies, and outliers in the financial data. Use automated data cleansing techniques to standardize formats, remove duplicates, and rectify data anomalies.
- Data Governance Framework: Carry out a robust data governance framework to confirm accountability and ownership of data quality processes. Define roles and responsibilities, establish data stewardship, and create policies and procedures for data management, data access, and data security.
- Data Integration and Consolidation: Ensure proper integration and consolidation of data from various sources to minimize data silos and inconsistencies. Implement data integration tools and techniques to streamline the data aggregation process and improve data accuracy.
- Regular Data Monitoring and Reporting: Institute ongoing data monitoring and reporting mechanisms to assess the quality of the financial data continuously. Employ data quality dashboards and reports to track data quality metrics and document any issues or trends that need attention.
- Data Quality Training and Awareness: Arrange for comprehensive employee data quality training. Foster a data-driven culture by promoting awareness of the importance of data quality and its impact on decision-making.
- Data Quality Measurement and Metrics: Define key performance indicators (KPIs) and metrics to measure the effectiveness of the data quality process. Regularly monitor and report on metrics to find areas of improvement and track progress.
- Continuous Improvement: Data quality is an ongoing process, so it’s essential to evaluate and continuously improve data quality initiatives. Regularly review and update data quality standards, refine validation rules, and incorporate feedback from data users to enhance the overall data quality process.
Maintaining high data quality is crucial for financial services organizations to safeguard customer satisfaction, avoid regulatory penalties, and protect the financial system. By implementing a comprehensive data quality strategy, these institutions can effectively decrease risks, enhance their decision-making processes, and ultimately drive business growth.
Now is the time for financial institutions to concentrate on data quality, invest in tools and processes, and foster a culture of data accuracy and integrity. A proactive approach ensures a more reliable and secure financial environment, paving the way for long-term success.
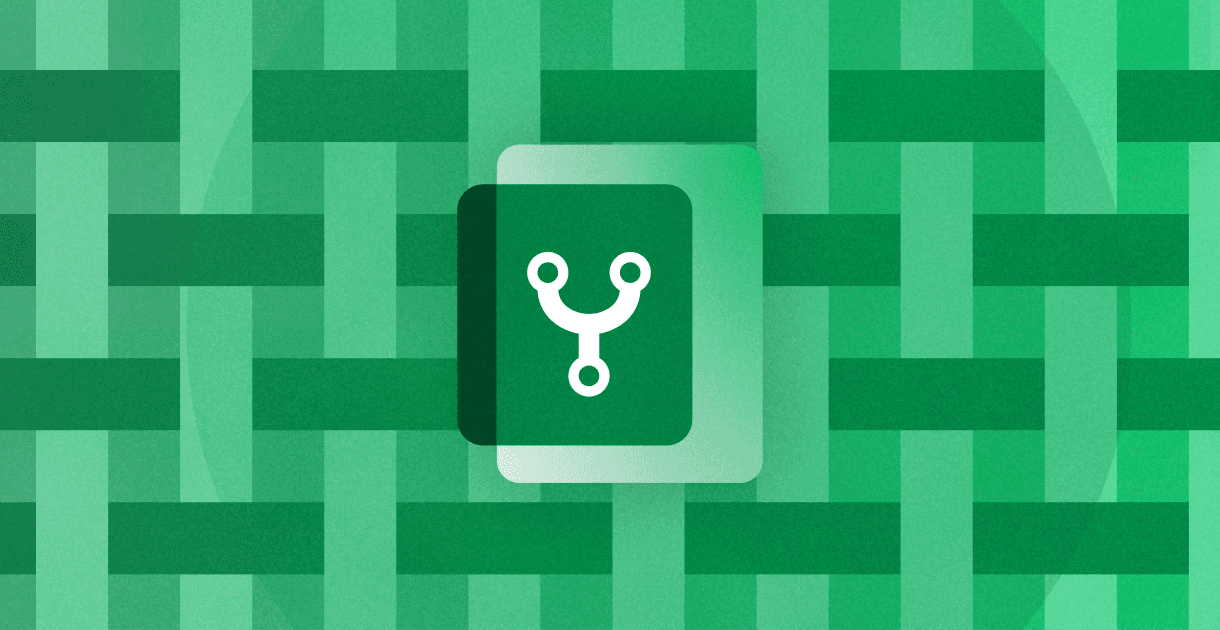
A Guide to Data Lineage
Data lineage is a pivotal aspect of data management, providing business users with the ability to understand and trace the journey of data from its original source to its final usage. By offering a transparent view of data’s lifecycle, data lineage ensures the reliability and quality of data, which is essential for making informed business […]
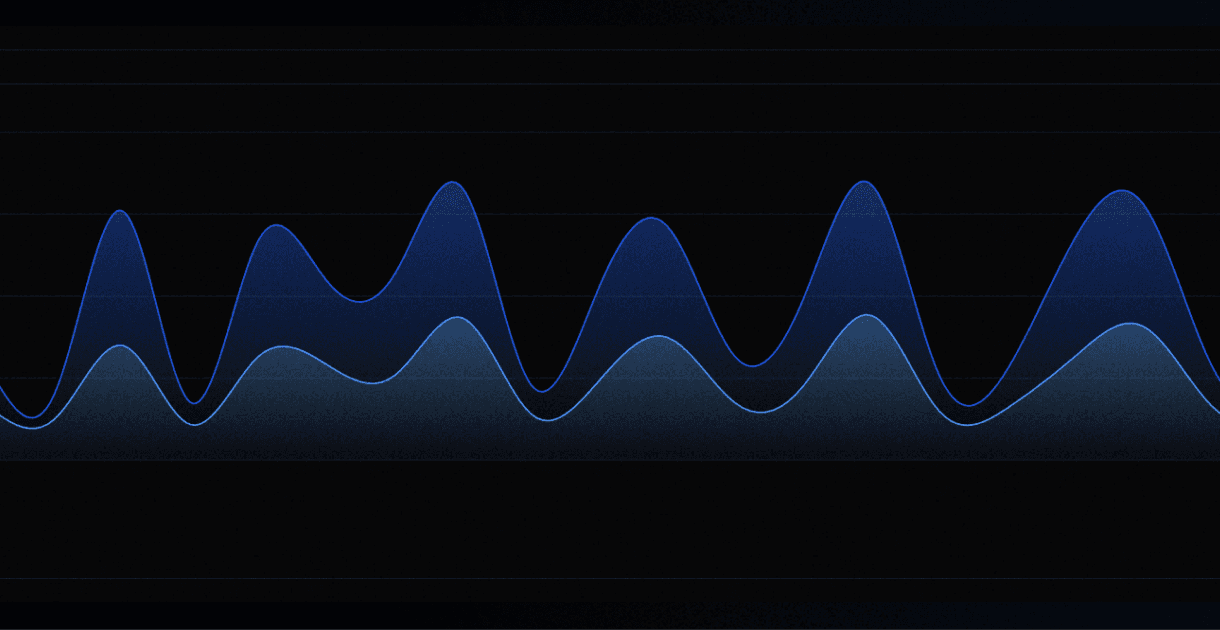
Understanding Postal Rate Changes
The cost of sending mail through the United States Postal Service (USPS) has been steadily rising for over a century, with few exceptions. For those tracking these changes over time, it’s clear that the mechanisms behind rate increases are complex and heavily influenced by legislative and economic factors. In this article, we will explore the […]